The University of California Berkeley Artificial Intelligence Research (BAIR)
Lab is pleased to announce the BAIR Open Research Commons, a new industrial
affiliate program launched to accelerate cutting-edge AI research. AI research
is advancing rapidly in both university and corporate research settings, with
existing collaborations already underway driven by individual
researcher-to-researcher collaborations. The BAIR Commons is designed to enhance
and streamline such collaborative cutting-edge research by students, faculty,
and corporate research scholars.
The Commons agreement has been framed with the goal of promoting open research
in AI: all on-campus effort, data, and results in the Commons program will be
non-exclusive with open publication and open-source code release expected.
Fostering an environment for excellence for graduate student research is the
primary motivation of the new program: Berkeley students will lead the design of
projects in the Commons, and the program of research must be approved by their
home departments before a project commences. Students are expected to benefit
from collaboration with leading researchers in industrial research labs, as well
as the availability of partner resources useful to investigate certain open
questions in state-of-the-art AI research. The University will benefit from
membership fees paid by partners to participate in the program. The Commons
agreement provides for collaborative joint projects between the partners and
Berkeley, with intellectual property shared jointly and equally by the parties.
The agreement also provides for joint research “lablets”, which will be embedded
collaborative open research spaces inside BAIR’s 27,000 sq. ft. research
facility opening this summer in the Berkeley Way West facility on the Berkeley
campus. More than a dozen faculty and 120 students will be assigned space in
the new lab, with an equal number of visiting positions allocated for
researchers from other BAIR labs and for visiting industrial partners.
Initial alliance participants include Amazon, Facebook, Google, Samsung, and
Wave Computing. Funding for over twenty joint projects has been committed in the
initial launch of the program, which will support both BAIR facilities and
research efforts. Over 30 faculty and 200 graduate students and postdocs at
Berkeley are affiliated with BAIR. For more information about BAIR or the
Commons program please contact bair-admin@berkeley.edu
.

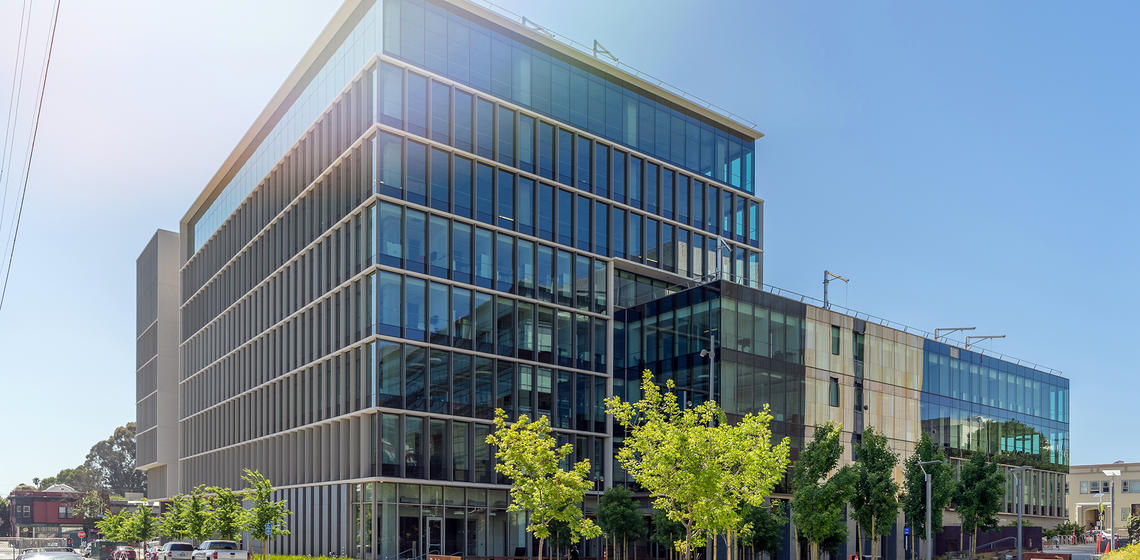
BAIR will occupy the top floor of Berkeley Way West.