Bumper Crop of AI Helps Farmers Whack Weeds, Pesticide Use
Weeds compete with neighboring crops for light, water and nutrients, costing the farming industry billions each year in agricultural yield.
To keep a better eye on fields, improve crop yields and reduce the use of pesticides, farmers and agriculture researchers are turning to AI.
“We believe the digital agriculture revolution will help in reducing the use of chemical products in agriculture,” said Adel Hafiane, an associate professor at the Institut National des Sciences Appliquées, in France’s Centre Val de Loire. Hafiane is working with colleagues from the University of Orléans to develop AI that detects weeds from drone images of beet, bean and spinach crops.
“If farmers can map the location of weeds,” he said, “they don’t need to spray chemical products over an entire field — they can just target specific areas, intervening at the right time and site.”
Using the georeferenced coordinates of where an aerial image was captured, farmers can determine the location of weeds in a field. The insights provided by the researchers’ deep learning network could then be deployed in agricultural robots on the ground that can remove or spray weeds in large fields.
Hafiane and his colleagues used a cluster of NVIDIA Quadro GPUs to train the neural networks. Their work was supported by France’s Centre-Val de Loire region.
Deep Learning on Cropped Images 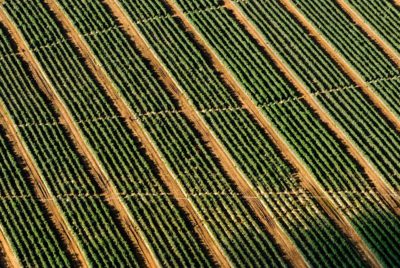
From a few hundred feet in the air, using low-resolution images, it’s not easy to tell the difference between weeds and crops — both are green and leafy. But with sufficient image resolution and enough training data, neural networks can learn to differentiate the two.
Using a dataset of tens of thousands of images for each crop (some labeled, some unlabeled), the team relied on transfer learning based on the popular ImageNet model to develop its deep learning models.
To partially automate the data labeling process, the researchers developed an algorithm that used geometric information in the images to label weeds and crops. Crops are often arranged in neat lines, with open patches of soil between the rows. When spots of green are visible in the space between crop rows, the AI knows it’s likely a weed.
A more complex challenge is detecting weeds within the crop rows. The researchers are working to improve their model’s results on spotting these trickier pests.
Developed using the TensorFlow and Caffe deep learning frameworks, the model recognizes weeds in fields of beets, spinach and beans. At a precision of 93 percent, the AI produced the best results analyzing beet crops.
Hafiane says using NVIDIA Quadro GPUs shrunk training time from one week on a high-end CPU down to a few hours. While the dataset used large, 36-megapixel images, the researchers say further increasing the image resolution captured by the drones would help boost the performance of their neural networks.
The researchers are also using NVIDIA GPUs to train neural networks to detect crop diseases in vineyards, and plan to collaborate with international colleagues to develop similar solutions to monitor other crops.
The post Bumper Crop of AI Helps Farmers Whack Weeds, Pesticide Use appeared first on The Official NVIDIA Blog.