Deep Learning Shakes Up Geologists’ Tools to Study Seismic Fault Systems
Fifteen years after a magnitude 9.1 earthquake and tsunami struck off the coast of Indonesia, killing more than 200,000 people in over a dozen countries, geologists are still working to understand the complex fault systems that run through Earth’s crust.
While major faults are easy for geologists to spot, these large features are connected to other, smaller faults and fractures in the rock. Identifying these smaller faults is painstaking, requiring weeks to study individual slices from a 3D image.
Researchers at the University of Texas at Austin are shaking up the process with deep learning models that identify geologic fault systems from 3D seismic images, saving scientists time and resources. The developers used NVIDIA GPUs and synthetic data to train neural networks that spot small, subtle faults typically missed by human interpreters.
Examining fault systems helps scientists to determine which seismic features are older than others and to study regions of interest like continental margins, where a continental plate meets an oceanic one.
Seismic analyses are also used in the energy sector to plan drilling and rigging activities to extract oil and natural gas, as well as the opposite process of carbon sequestration — injecting carbon dioxide back into the ground to mitigate the effects of climate change.
“Deep learning isn’t just a little bit more accurate — it’s on a whole different level both in accuracy and efficiency.” – Sergey Fomel
“Sometimes you want to drill into the fractures, and sometimes you want to stay away from them,” said Sergey Fomel, geological sciences professor at UT Austin. “But in either case, you need to know where they are.”
Tracing Cracks in Earth’s Upper Crust
Seismic fault systems are so complex that researchers analyzing real-world data by hand miss some of the finer cracks and fissures connected to a major fault. As a result, a deep learning model trained on human-annotated datasets will also miss these smaller fractures.
To get around this limitation, the researchers created synthetic data of seismic faults. Using synthetic data meant the scientists already knew the location of each major and minor fault in the dataset. This ground-truth baseline enabled them to train an AI model that surpasses the accuracy of manual labeling.
The team’s deep learning model parses 3D volumetric data to determine the probability that there’s a fault at every pixel within the image. Geologists can then go through the regions the neural network has flagged as having a high probability of faults present to conduct their analyses.
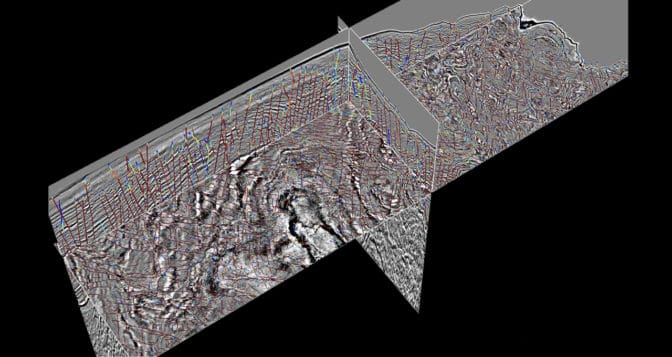
“Geologists help explain what happened throughout the history of geologic time,” he said. “They still need to analyze the AI model’s results to create the story, but we want to relieve them from the labor of trying to pick these features out manually. It’s not the best use of geologists’ time.”
Fomel said it can take up to a month to analyze by hand fault systems that take just seconds to process with the team’s CNN-based model, using an NVIDIA GPU for inference. Previous automated methods took hours and were much less accurate.
“Deep learning isn’t just a little bit more accurate — it’s on a whole different level both in accuracy and efficiency,” Fomel said. “It’s a game changer in terms of automatic interpretation.”
The researchers trained their neural networks on the Texas Advanced Computing Center’s Maverick2 system, powered by NVIDIA GPUs. Their deep learning models were built using the PyTorch and TensorFlow deep learning frameworks, as well as the Madagascar software package for geophysical data analysis.
Besides faults, these algorithms can be used to detect other features geologists examine, including salt bodies, sedimentary layers and channels. The researchers are also designing neural networks to calculate relative geologic time from seismic data — a measure that gives scientists detailed information about geologic structures.
The post Deep Learning Shakes Up Geologists’ Tools to Study Seismic Fault Systems appeared first on The Official NVIDIA Blog.